Quick Read
In the Race to Adopt AI, Your Data Is the Advantage
Transform your data and context into a true AI advantage
May 01, 2025
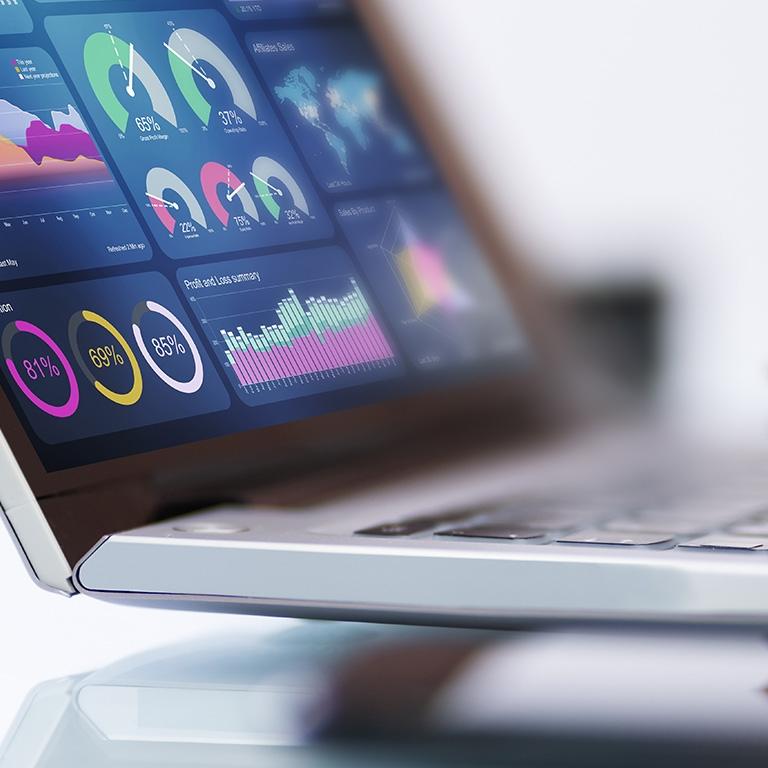
In the Race to Adopt AI, Your Data Is the Advantage
AI models and enterprise integrations are rapidly becoming commodities that are accessible to nearly everyone. But real competitive advantage doesn’t lie in the model itself. It lies in the data that fuels it.
For CIOs and CDOs, the critical question is this: Are the most valuable AI models built on publicly available data, proprietary data, or a hybrid of both?
The answer: Proprietary and domain-specific data—combined with the right context—will drive the most meaningful and measurable impact.
What differentiates AI efforts today isn’t power. It’s precision.
While large language models (LLMs) continue to evolve, we’re seeing diminishing returns in performance alone. Modest differences between models won’t matter nearly as much as how—and where—you apply them.
The difference-maker? Context-rich, trustworthy data that reflects your business and industry.
Consider how this plays out across sectors:
- In healthcare, the most valuable insights come from internal patient records and claims—not generalized medical datasets.
- In manufacturing, proprietary operational and equipment data trains machine learning models that can anticipate disruptions and boost efficiency.
- In financial services, years of transactional data power predictive risk and fraud models that public sources can’t match.
In recent years, we’ve also seen organizations mature in how they govern their data—showing increased rigor in reviewing data contracts with third parties to protect their competitive data assets. Organizations are recognizing that their proprietary data is a core strategic asset that requires both defensive protection and proactive management.
This is where proprietary and hybrid datasets come in—offering unmatched depth and relevance when applied with domain knowledge.
Context is king. And governance is what makes it usable.
Your ability to extract meaning from data is strengthened by domain expertise. You understand the relationships between data points in ways a generic model simply can’t.
That’s why organizations must take an active stance in protecting their data and IP—whether that means preventing scraping from their websites, reviewing data-sharing contracts with partners, or implementing guardrails around how agents and bots can interact with their platforms.
But even the most context-rich dataset is only as valuable as it is governed. That’s why organizations are accelerating their investment in:
- Data management to clean, label, and prepare AI-ready data
- Governance frameworks to ensure quality, trust, and compliance
- Access strategies that balance usability with security
In the classical world of AI and machine learning, clean tabular data—structured in databases—was the gold standard. Now, with the rise of GenAI, that definition is expanding. Organizations must manage and enhance unstructured data like documents, audio, and video with the same intention and rigor. This unstructured data is increasingly foundational for GenAI use cases.
We’re also talking more about the intersection between the physical and digital worlds. Capturing data at the edge—through video on retail floors, computer vision on shop floors, or sensor data in automotive environments—is becoming a top priority. This data, though often messy, is immensely valuable when structured and governed appropriately.
These foundational efforts not only reduce risk but also enable smarter, faster AI experimentation—because the right data is where it needs to be, when it needs to be there.
Leading vendors aren’t just building AI—they’re fueling it with the right data
Companies winning in the AI space are pairing infrastructure investments with proprietary data strategies. Consider how a few leaders are pulling ahead:
- Databricks is enhancing “talk with your data” use cases through Unity Catalog’s rich metadata—bringing clarity to governance and access at scale.
- Salesforce is unlocking autonomous agents through Agentforce, made possible by its clean, structured customer data.
- ServiceNow is modernizing omnichannel support and IT workflows by applying AI to well-governed operational data.
Why are these vendors leading? Because they’re applying GenAI to the most differentiated data they own—metadata, service interactions, ticketing workflows, and more. Look at where the most compelling GenAI applications are happening: It’s with the vendors who have the most unique, domain-specific datasets.
So where should you invest?
To compete—and win—in the AI-enabled future, organizations must focus on three critical areas:
- Build domain-specific applications that apply AI to the problems your teams know best.
- Leverage proprietary and hybrid datasets that reflect your unique business dynamics.
- Create connected data ecosystems that support seamless access, collaboration, and governance across departments.
That also means expanding the value of your data. Organizations should assess what data they’re capturing (or letting slip away), invest in improving data quality, tighten entry and cleanup processes, and look for opportunities to enhance internal data with curated third-party sources—whether purchased, scraped, or bartered with trusted partners.
Authors: Erik Brown and Cameron Cross