Quick Read
Understanding bias in AI: Beyond the headlines and hype
Get actionable steps to tackle bias in AI, such as scrutinizing data, investigating algorithms for potential bias sources, and diversifying your team to ensure various perspectives.
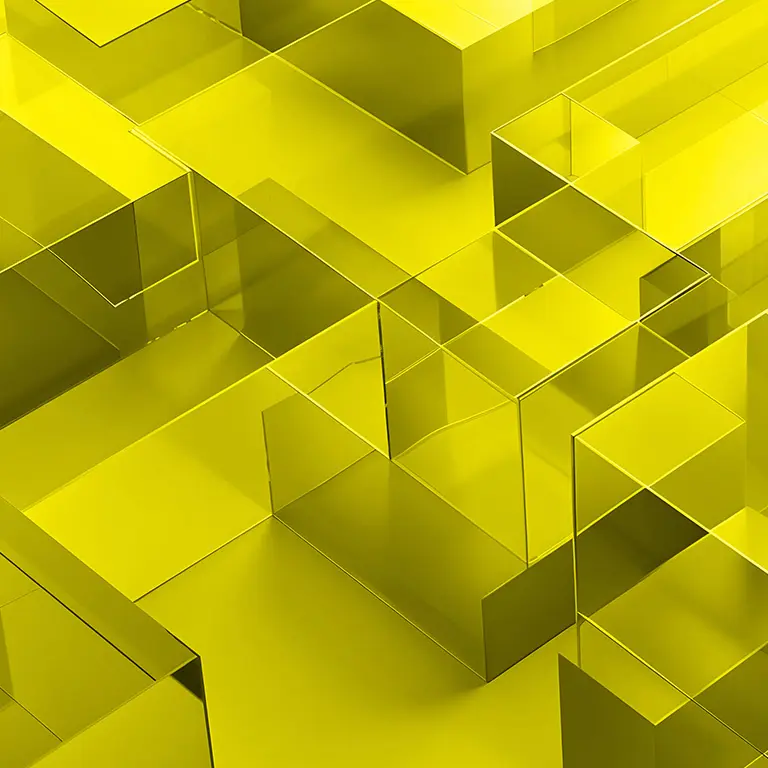
AI and ML technologies are making waves all around us—from ChatGPT in classrooms to potential disruptions in white-collar jobs. As the conversation keeps buzzing with new advancements and controversies, it's crucial to examine the ethical implications of bias lurking within algorithms, data sources, and machine learning models. This hidden bias can significantly impact cultural, racial, gender, industry, history, and scientific perspectives. We’ll delve into ways to ensure ethical AI and ML technology use and minimize the effects of bias.
Unraveling bias in AI: Examples and where it comes from
Before tackling bias in AI and ML models, it's vital to understand some of its sources. While not an exhaustive list, some common origins involve biased data, biased algorithms, and biased results interpretation. Each of these sources can impact the fairness and effectiveness of AI and ML applications, and it's crucial to understand them in detail.
Biased data occurs when the training dataset isn't representative of the desired result or insight the model presents. Various reasons can lead to this situation, including underrepresentation or overrepresentation of certain demographic groups, data collection methods that inadvertently favor specific segments of the population, and outdated or historically biased information used as the basis for the training dataset.
One example of bias in AI: Underrepresentation of women and minority groups in a facial recognition system's training data could lead to higher error rates for these groups. In a healthcare-related example, a diagnostic algorithm might inadvertently favor certain segments of the population if the data collection method relies primarily on medical records from a specific demographic, potentially excluding people with different health backgrounds or conditions that are more prevalent in underrepresented communities. Using historical data to train a predictive policing algorithm also may result in perpetuating biased law enforcement practices because the dataset may reflect the disproportionate targeting of specific communities. Addressing biased data is essential for building AI and ML models that are fair, accurate, and applicable to diverse populations.
Biased algorithms arise when the model is designed to prioritize specific attributes, resulting in unfair treatment of certain groups or unintended/unconsidered consequences. This can happen due to unintentional encoding of human biases into the algorithms by developers (like an individual’s preferences), overemphasis or underemphasis of certain features that lead to skewed predictions or recommendations, and a lack of awareness or understanding of how various attributes may interact and influence the model's outcomes and the interpretation of the model’s insights. Recognizing and addressing biased algorithms is crucial for creating AI and ML models that can make unbiased decisions or predictions.
Biased interpretation occurs when the model's results aren't fairly and objectively assessed. This can manifest in several ways, including confirmation bias where the interpreter focuses on results that confirm their preconceived beliefs while ignoring contradicting information. For example, in AI-driven hiring systems, an employer might only pay attention to candidates with traditional backgrounds, reinforcing their belief that non-traditional candidates are not a good fit. Similarly, in medical diagnostics, a doctor might give more weight to an AI's diagnosis that aligns with their initial thoughts, disregarding alternative possibilities suggested by the system. Misinterpretation of the model's outputs can potentially lead to incorrect conclusions or actions, and a lack of transparency regarding the model's limitations, assumptions, or potential biases may lead to overconfidence in the results.
Understanding the roots of bias is crucial when tackling fairness in AI and ML systems. While biased data, biased algorithms, and biased results interpretation are common sources, there are numerous other examples and origins of bias. To deepen your knowledge and uncover additional bias sources, consider exploring academic research, attending workshops or webinars, or engaging with AI ethics communities. By staying informed and proactive, you can contribute to a more equitable AI and ML landscape.
In the meantime, let’s talk about some steps you can take right now to address bias in your AI and ML applications.
Tackling bias in AI and ML: Actionable steps for ethical implementation
Step 1: Examine data for bias
To certify that your data isn't biased, scrutinize it for potential bias sources per the definition above. You can use tools like IBM's AI Fairness 360 to identify and mitigate dataset bias. Additionally, ensure your data represents the studied population by gathering data from diverse sources and using proper sampling techniques.
Step 2: Inspect algorithms for bias
To guarantee unbiased algorithms, investigate them for potential bias sources. Utilize tools like Google's What-If Tool to visualize how different inputs impact your model's output. Techniques like adversarial training—intentionally introducing biased data into your model—can help make your model more resilient to bias.
Step 3: Fair Results Interpretation
For fair results interpretation, avoid basing your model's results on preconceived notions or biases. Be transparent about your methodology and potential bias sources. Embrace feedback and criticism, and be prepared to adjust your model or results interpretation as needed.
Step 4: Bring Diversity to Your Team
To make your AI and ML projects inclusive and fair, diversify your team. This ensures various perspectives in model development and helps identify and address potential bias sources. Consult with experts in sociology, cultural anthropology, and ethics for a deeper understanding of cultural, racial, and gender bias impacts.
In conclusion
Promoting ethical AI and ML technology use and curbing bias is essential for creating inclusive and fair experiences for everyone. By following the steps outlined in this blog post, you can start to identify and address potential bias sources in your data, algorithms, and results interpretation. Start by exploring the tools and resources mentioned, and consult with field experts. Build your expertise in data science. Create a culture of hypothesis, test, and verify that is open to being wrong and making changes to improve your solutions. Together, we can strive for a fairer and more inclusive AI and ML future.
Authored by: Eric Johnson