Guide
Demystifying AI and ML for Customer Support Operations and Delivery
AI and machine learning solutions are emerging as a critical component for the digital strategies that support leaders are implementing to streamline operations—but questions still linger
May 02, 2023
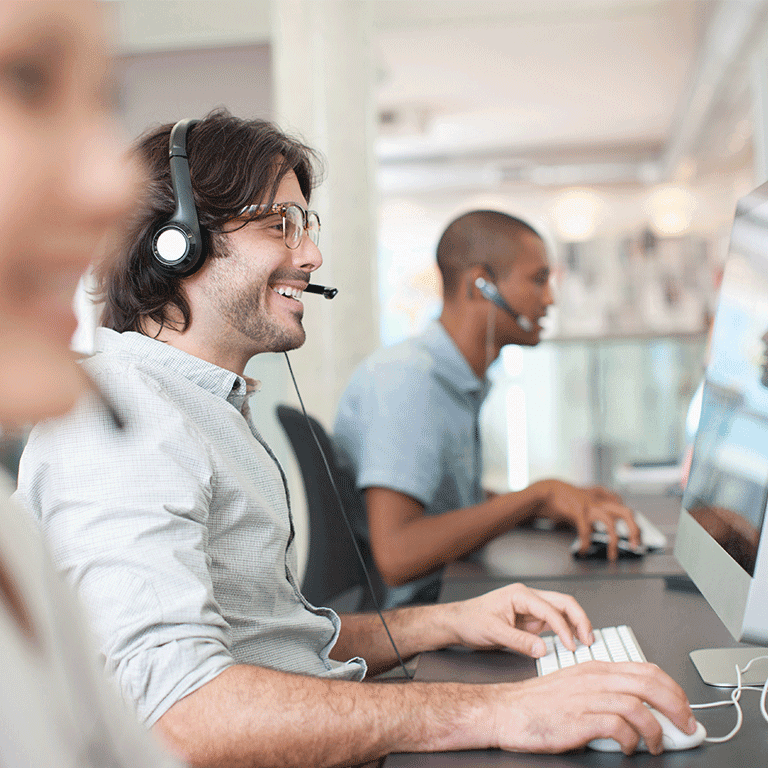
“The goal of a customer support organization is to go out of business.”
This tongue-in-cheek refrain in the customer support community teases at the dependency software providers have on support delivery given product complexity and immaturity.
The mandate of customer support has always been to balance the customer experience with efficient, scaled operations in the context of the product roadmap and known gaps. But this quip feels particularly relevant in the recent environment—shrinking budgets and economic headwinds increasingly pressure customer support operations to do more, to do it faster, and to do it with less.
Faced with this pressure, support leaders have been earnestly solutioning an evolved set of questions:
- How can I bend the curve on case volumes and reduce costs per case?
- How can I speed up time to resolution while improving quality of resolution?
- How do I get my teams up the knowledge curve quickly and efficiently?
- How can I do all of this while enhancing the customer experience?
These questions have laid the groundwork for the digital strategies that leaders are actively implementing today—and Artificial Intelligence (AI) and Machine Learning (ML) solutions have already emerged as a critical component.
Support organizations are actively experimenting with these capabilities as a new pathway to scalable growth. The resulting vision of a low or “no-touch” support model fueled by knowledge and training, continuous and comprehensive analytics, and intelligent workflows is palpable—not because products have grown simpler to use, but because of the promise of automation, AI, and ML in streamlining support operations.
Far less clear, however, is the roadmap on where to begin and how to utilize these capabilities quickly and effectively. That’s where we come in.
Frame your AI/ML journey by asking the right questions
Maturity of usage today is varied. Many are already leveraging AI and ML, some are “waiting and watching” to better understand the leading tools and overall limitations, and others are planning to get started in the not-too-distant future.
But in all cases, it’s easy to be swept up by the constant barrage of AI in the news and feel acute pressure to pick some tools and rapidly implement AI/ML.
It’s prudent—as is the case with any large-scale transformation—to carefully consider what AI will (and will not) do, where it can make the most impact, and the implications involved in deploying it within your digital strategy.
These are just some of the potential questions to consider as you craft your own AI journey:
What will be the overall impact on my customers' experience? My employees and their jobs? My business? These tools should not be seen as single-threaded solutions to cut headcount, increase self-service, or analyze customer interactions. There’s a fundamental re-definition of the way that employees, customers, and tools work together, train, and enrich each other.
For example, employees can be freed of repetitive, manual tasks to focus on the highest-value activities for customers. Jobs, customer expectations, and even how we view our business will fundamentally change, making way for the emergence of new skills, employee requirements and customer deliverables.Is the technology mature enough? What are security, ethics, and privacy implications? As with any disruption, it’s reasonable to question whether downstream implications and risks are well understood—especially without clear regulatory direction. Consider how existing controls and compliance processes will interact with the new workflow and how threats might surface by instituting these tools. Understand your risk tolerance and what that means for your pace of adoption.
What about my data and infrastructure? Data is perhaps the most foundational aspect to properly leveraging these tools. If your data quality, governance, taxonomy, and systems are immature, you will not be able to fully take advantage of all that AI has to offer. Think critically about your tech stack, tech debt, and internal teams managing this. How big of a lift will it be to feed quality data into the tool and manage it? How much of a challenge has it been to integrate other tools into existing infrastructure?
Begin your AI/ML journey with practical guidance
Though it may require sifting through a number of options and considerations, there are a few best practices and steps that organizations can take.
Begin with the use case and the underlying business need driving it
Don’t simply jump to tool selection as a way to quickly get AI into the workflow. Instead, focus on matching the use cases that AI/ML can optimize to your metrics in need. As an example, if your financials are great but you face low support NPS/CSAT scores, adding an AI chatbot as the first line of defense might not be right step.
Embrace a mindset of rapid experimentation
Speed and intelligence should be viewed as parallel priorities. Be smart and structured in your approach—but move quickly. For organizations with more of an appetite for risk, this is an opportunity to embrace a “fail fast” approach. Test several different tools and learn from your experience as opposed to making a massive investment in one tool—just to find out it wasn’t the right fit. Taking the time to determine the right combination of tools can save time, money, and will help ensure the right foundations are in place.
Listen to your customers and employees
Regardless of the tool you decide to implement, ensure that it enhances the customer experience. It’s critical that productivity gains are not negated by customer dissatisfaction and churn. You also must keep a pulse on your employees; while new tools might seek to reduce and automate processes, they must learn how to properly use and adapt to them. Tech adoption can cause anxiety for employees, especially in roles where new solutions can be seen as “replacing” fundamental parts of their job function. It will be essential to help your teams understand how their roles are changing to manage attrition risk and generate adoption.
Be prepared for short term uncertainty
As you implement new technology, it’s prudent to be prepared for short-term disruption. Productivity losses are often associated with implementation, learning and adoption to the new technology, and fear-based attrition. It’s also common to experience operational metrics change or in unexpected ways. For example, as you increasingly deflect support inquiries through smarter and wider delivery of relevant self-service content, you may find the overall complexity of incoming cases growing, increasing case costs and resolution. Depending on the direction you take, it may require some time to find your new normal.
Where we’re seeing adoption of AI/ML in customer support
While there’s a universe of use cases out there today, certain common entry points seem to have emerged for enterprise software providers.
AI-powered chatbots to streamline customer engagement
One of the more common examples—especially with the recent focus of generative AI solutions on natural language processing tools—is chatbots. Correctly implementing chatbots can provide customers with quick and accurate responses to their inquiries and lead to reduced response times.
Leverage robotic process automation (RPA) to automate repetitive tasks
RPA can be used to automate routine, repetitive tasks such as data entry, form filling, data retrieval, material development, and even certain cursory analysis. This can reduce the manual burden of customer-facing and internal tasks and can help bridge gaps in disparate/immature data structures for reporting and analysis.
Analyze more of your available customer data
Customer emails/phone calls and customer communities represent one of the greatest untapped sources of insight. Community analytics and sentiment analysis can be used to monitor and identify trends in customer feedback. This data can then be used to identify areas of improvement for products and services, leading to improved customer satisfaction.
Predict escalations, churn, and provide better insights to customers
The right tech solutions can be used to identify issues that are likely to escalate, enabling proactive customer support to address issues before they become critical (and over time, to address questions before they even become issues). The same processes can be used to provide better guidance and visibility to software users to better optimize their experience and usage. The notion of support teams going out of business in favor of no-touch delivery is (probably) not meant to be taken seriously. But as support organizations seek to harness the power of AI and ML to better balance the customer experience, it’s likely that delivery models, metrics, and jobs will fundamentally change. Leveraging some of these tactics to begin implementing and utilizing AI and ML will help you understand how it best fits in within your support organization and prepare you for inevitable changes to come.